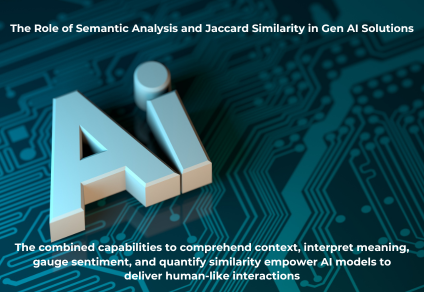
Role of Semantic Analysis and Jaccard Similarity in Gen AI Solutions
_______ Sankar SanthanaramanIn the age of AI, particularly in the generation of sophisticated AI models, semantic analysis & Jaccard similarity play pivotal roles in unlocking the true potential of natural language processing (NLP). Semantic analysis, a branch of NLP, goes beyond mere language understanding, delving into the intricate nuances of meaning, context & relationships within textual data. Jaccard similarity, on the other hand, contributes a quantitative measure to assess the similarity between sets of words.
At its core, semantic analysis aims to comprehend the intended meaning behind words & phrases, bridging the gap between human communication and machine understanding. In the context of developing Generative AI (Gen AI) solutions, semantic analysis becomes an indispensable tool, offering several key advantages.
One of the primary benefits lies in the contextual interpretation of language. Gen-AI systems equipped with robust semantic analysis capabilities can discern the contextual variations of words, ensuring a more accurate & nuanced comprehension of user inputs. This nuanced understanding is crucial for generating human-like responses, making interactions with AI models more natural and effective.
Jaccard similarity, a measure of the similarity between two sets, finds relevance in the context of semantic analysis. By quantifying the overlap between sets of words, jaccard similarity provides a valuable metric for assessing the similarity of documents or phrases. In Gen AI, this measure can enhance the accuracy of semantic analysis by quantifying the semantic similarity between user queries and stored knowledge.
Moreover, semantic analysis contributes to improved information retrieval. By grasping the underlying meaning of queries, Gen AI systems can retrieve relevant information more effectively, offering users precise & contextually appropriate responses. This not only enhances the user experience but also boosts the overall efficiency of AI-driven applications.
Another critical aspect is sentiment analysis, an integral component of semantic analysis. Gen AI solutions, with the ability to decipher sentiment from text, can tailor responses based on emotional context. This emotional intelligence, combined with Jaccard similarity, adds a layer of sophistication to AI interactions, fostering more meaningful and empathetic exchanges.
In conclusion, semantic analysis and Jaccard similarity serve as the cornerstones for the evolution of Gen AI solutions. Their combined capabilities to comprehend context, interpret meaning, gauge sentiment, and quantify similarity empower AI models to deliver human-like interactions. As we continue to advance in AI development, the integration of semantic analysis and Jaccard similarity stands as a testament to our commitment to creating AI systems that not only understand language but truly grasp the intricacies of human communication.